Random Selection and Random Assignment in Educational Research
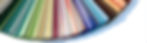
In designing a quantitative research project where statistical analysis will provide the basis for inferences made about the data collected, a reliable and valid method of sample selection is vital. As part of the initial design process, a researcher will determine the independent and dependent variables of the study (that is, the experimental and response variables) and then identify a target population. From that target population, observations and experimentation will yield data that will either allow or cause the failure of the rejection of the null hypothesis through the application of appropriate statistical procedures. For this reason, while every element in the design of a research study contributes to its success, none of them can compensate for the damage done by bad data obtained from erroneous or improper sample selection (Scott & Morrison, 2007). With that said, let's explore random sampling as a foundational component of the design process in quantitative research within the context of a hypothetical study that would infer the likelihood of the passage of an educational bond referendum.
A population is some whole and distinct group of items or subjects from which a sample is drawn (DeMoulin & Kritsonis, 2013). The population pool may be as large as a city or even a nation or as small as a classroom of students. It may be comprised of people or items, though in the social sciences, the former almost universally constitutes the populations of interest (Eldredge et al., 2014). The deciding factor for identifying a population is typically a characteristic the items or subjects have in common and the concern a researcher has for studying that characteristic. In addition to identification, researchers must further determine the accessibility of any target population. While the accessible population may be the same as the target population, it may not. In the theoretical education bond study for this paper, the target population is all eligible members of a voting district; this is also the accessible population (available through census and voter registration records). Suppose, however, that one were investigating student demographics and academic performance within a district, but one or more of its school principals declined to participate. The result would be an accessible population smaller and different from the target population of all students in a given district (DeMoulin & Kritsonis, 2013).
Because the study of a whole population can be prohibitive to a researcher’s work, either because of funding and resource limitations or simple impracticality, data are typically collected from samples (Vogt & Johnson, 2015). A sample is a subset of a population. For a quantitative study, where a researcher will apply statistical analysis to the data, this sample is assumed to be random. True-random samples are representative of a population and allow for inferences to be generalized from the sample to the population. Those inferences should not be viewed as truth claims, however; instead, they are probabilistic descriptions, correlations, and explanations of phenomena observed within the sample. For a sample to be true-random, the method of selection must meet two criteria. First, that each item or subject within the sample has an equal probability of being drawn; and second, that each item or subject is drawn independently and not affected by the drawing of any other one (Suhonen et al., 2015). When created in this way, a sample will be representative of the population, and a researcher may subject the empirical data collected from it to parametric statistical procedures.
In practice, to create the actual sample from a population, a researcher needs first to create a sampling frame. This is a list, typically in the form of a database, that contains all members of the target population. Even a basic statistical software package like Excel is able to assign identification numbers at random to all items or subjects within a population list. The researcher need only provide the required sample size (n) and use the software to apply random number generation to the set based on the total population (N). If a sample size of 500 is required, one simply draws the randomly assigned numbers one through five hundred from the total population database. Note, however, that just as there is an important distinction between the target population and accessible population that will determine the true number or N of a study, there is similarly an important distinction between sample selection and the actual sample number or n. The actual sample number is the number of items or subjects from which data will have successfully been collected. Even if in the educational bond study, a true-random sample of 500 subjects were drawn, should only 430 of them provide data for the study, the actual sample would be n = 430 and not n = 500.
Generating a Stratified Random Sample
Stratified sampling is a method of random sample selection that first divides a population into smaller subsets referred to as strata (Scott and Morrison, 2006). Within each stratum, a researcher can draw an individual, random sample. The strata, which a researcher identifies in advance, create sub-categories for the data that allow statistical inferences within those specific categories. These sub-categories express within-group homogeneity and between-group heterogeneity and are identified to increase the precision of sample representation. Such sampling may also contribute to the study’s internal validity by identifying and deliberately construing potential confounding variables (Vogt and Johnson, 2015). In the social sciences, these categories typically align with demographics (age, race, gender, sex, SES, etc.) or psychological features (facets of child development, personality, and mental health). Random number generation as described above using Excel or a similar software package is again used in the creation of a sample, though this process assumes that the categories used in the strata are available to the researcher in database form in advance.
To study the question of whether a population is likely or not to support the passage of an educational bond, stratification related to demographics could inform decision-making for canvassing and other informational campaigns. Data collected on the basis of categories such as gender, age, and parental status (which is to say, whether or not the respondents have children, and whether those children attend a public school in the voting district) would enhance understanding not only of whether the bond might pass, but also of what categories of individuals are likely to support or not. Parental status is a particularly useful category in this instance, in my view, because I suspect that the likelihood of supporting or not supporting a bond will correlate with whether households have children and whether those children attend public schools in the voting district. Knowledge of correlations between categories of data would allow door-to-door canvassers and informational campaigns (local television commercials and brochures) to target specific types of individuals with likely known viewpoints. It may become clear from the study that individuals without children currently attending a public school in the district, for instance, are unlikely to support the bond’s passage. Such information is valuable in generating an argument either for or against the bond measure, depending on who are the information users of the study. Whoever the information users, though, a stratified sample will allow canvassers and campaign communications to target their arguments more effectively to their audiences.
Generating a Cluster Random Sample
Cluster sampling, like stratified sampling, is a random form of sample section in which a researcher divides a population into smaller groupings referred to as clusters (Scott & Morrisons, 2006). Individual samples are randomly drawn from each cluster. Like stratified sampling, cluster sampling is a probabilistic and random method, but expresses between-group homogeneity and within-group heterogeneity. As discussed above, the purpose of stratified sampling is to identify categories like demographics explicitly and organize sample selection using population groups defined by these categories; hence, the within-group homogeneity. Cluster sampling, by comparison, does not use researcher-defined categories, but instead divides a population by natural features like geography. Within a voting district, clusters are inherently created by street intersections and neighborhoods. For this reason, there is within-group heterogeneity (that is, demographic traits like age and parental status have not been identified), but between-group homogeneity (that is, each cluster is equally a neighborhood). The primary reasons that a researcher would use cluster sampling are to reduce the overall cost of a study and increase efficiency. Once again, population lists are a prerequisite for random selection of the sample using a statistical software package.
Conclusion
Fundamental to the success of a quantitative research study is the method used for sample selection. The data for a study come from the observations of and treatments applied to a sample. Should that sample not have been selected correctly, the data coming from it will be unreliable and invalid. Such data cannot produce results that allow meaningful inferences about a phenomenon of interest to a researcher and cannot be generalized to a population. In other words, if the sample is poor, the data will be poor, and the study will produce erroneous or, at best, low-quality results.
The process of sample selection is a necessary early step in the design of a quantitative research study. To apply parametric statistical procedures to data and make generalizations from the sample to the population requires that the sample selection be true-random and, thus, representative. This means that each item or subject within the sample has an equal probability of selection and that each one is also independent of the others. In the social sciences, stratified and cluster sampling are common methods of sample selection that allow a researcher, based on constraints of time and funding, either to increase representation while decreasing potential confounders (as with stratification) or to reduce costs while improving efficiency (as with clustering). Because there is no curative in the design of a research study for the invalid and unreliable results that bad data produce, a systematic approach to sampling should include the following steps:
Identification of a target population to address the variables of the research question.
A decision of needed sample size which, in connection with effect size and p-value will determine the power of the study as a whole. (Notably, sample size is the most commonly manipulated component of a study’s power as effect size and p-value are typically designated in advance and used to determine the required sample size)
Based upon the identified sampling method (for instance, simple random, random clustering, or random stratification), a statistical software package is used to generate the random sample from a population database.
The researcher proceeds with data collection.